Xiaomi MiMo AI
|Xiaomi introduced the groundbreaking MiMo reasoning-focused AI model to the world as an open-source initiative, marking a significant shift in the landscape of reasoning models. Known as MiMo, this family of reasoning models is designed to revolutionize the optimization of reasoning capability within a compact parameter size, a feat unseen before in the tech giant’s repertoire. This blog discusses about the Xiaomi MiMo AI.
Key Highlights
This release marks Xiaomi’s maiden entry into the realm of open-source reasoning models, as it sets up a competition with its Chinese counterparts like DeepSeek R1 and Alibaba’s Qwen QwQ-32B, along with global models such as OpenAI’s o1 and Google’s Gemini 2.0 Flash Thinking. One of the key highlights of the MiMo series is its inclusion of four distinct models, each tailored for specific and unique use cases, showcasing the diversity and versatility embedded within Xiaomi’s AI innovation.
Salient Aspects
The genesis of the MiMo project stems from Xiaomi’s researchers, who harbored the ambition to tackle the prevalent issue of size scalability in reasoning AI models. Traditional reasoning models have been characterized by their staggering parameter count, often featuring at least 24 billion or more parameters. Xiaomi’s MiMo models depart from this norm by boasting a relatively compact seven billion parameters, a deliberate effort to strike a balance between coding prowess and mathematical proficiency, an endeavor typically challenging to achieve with larger models. This article enumerates about the Xiaomi MiMo AI.
Model’s Development Journey
Xiaomi boldly claims that MiMo’s performance stands shoulder to shoulder with the likes of OpenAI’s o1-mini, even managing to surpass other reasoning models equipped with a staggering 32 billion parameters. Such remarkable efficacy, according to the researchers, is a result of meticulous optimization strategies employed throughout the model’s development journey. From streamlining data preprocessing pipelines to refining text extraction toolkits and implementing sophisticated multidimensional data filtering mechanisms, every aspect of MiMo has been meticulously crafted to deliver unparalleled reasoning prowess.
Mixture Strategy
Furthermore, MiMo’s pre-training methodology underscores a three-stage data mixture strategy, a meticulous process engineered to enhance the model’s adaptability and performance. Internal testing results have been promising, with the MiMo-7B-Base model clocking an impressive score of 75.2 on the BIG-Bench Hard (BBH) reasoning benchmark, a testament to its exceptional capabilities. Another variant, the MiMo-7B-RL-Zero, harnesses zero-shot reinforcement learning techniques to excel in mathematical and coding-related tasks, boasting a commendable 55.4 score on the AIME benchmark, surpassing the performance of OpenAI’s o1-mini by a notable margin of 4.7 points.
Summary
For the broader AI community, the availability of MiMo as an open-source model represents a pivotal moment, as it allows enthusiasts and researchers to delve into its intricacies. Interested parties can access the model through Xiaomi’s GitHub and Hugging Face repositories, granting unprecedented access to its architecture, pre-training processes, and post-training techniques. While MiMo excels in the realm of text-based tasks, it currently lacks multimodal capabilities, a clear distinction that sets it apart from its contemporaries. Yet, in the spirit of transparency and collaboration that defines open-source projects, crucial details about the model’s dataset remain obscured, leaving room for further exploration and investigative endeavors within the AI community.
LEAD GENERATION SERVICES FROM APPSREAD

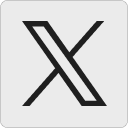




