DeepSeek Prover V2
|DeepSeek, the Hangzhou, China-based artificial intelligence (AI) firm, recently introduced an enhanced version of its specialized Prover model, named DeepSeek Prover V2, which zeros in on proving formal mathematical theorems. Making waves in the AI community, this large language model (LLM) adeptly utilizes the Lean 4 programming language to meticulously scrutinize the logic of mathematical proofs, scrutinizing each step independently to ensure impeccable accuracy.
DeepSeek’s New Mathematics-Focused AI Model Is Here
The release of DeepSeek’s mathematical powerhouse, DeepSeek-Prover-V2, was met with enthusiastic reception, especially within the mathematical domain where it promises groundbreaking advancements. Disclosed on the firm’s GitHub listing page, this innovative model serves as a testament to DeepSeek’s commitment to innovative thinking and technological progress. Drawing from the groundwork laid down by its predecessor, DeepSeek-V3, which made its debut in December 2024, the Prover-V2 model boasts a refined chain-of-thought (CoT) specifically tailored to tackle mathematical challenges head-on.
Standout Feature
The versatility of DeepSeek-Prover-V2 is a standout feature, offering a wide array of functionalities that cater to various users. From facilitating the resolution of high-school to college-level mathematical problems to enhancing the accuracy of theorem proofs, this model proves to be a valuable asset for educators, mathematicians, and researchers alike. Furthermore, it stands as a powerful tool for generating detailed explanations of proofs in a step-by-step manner, fostering enhanced understanding and analysis of complex mathematical concepts.
Noteworthy is the option to choose between two model sizes — a compact seven billion parameter size and a more robust 671 billion parameter size. While the former is based on DeepSeek-Prover-V1.5-Base, the latter draws upon the foundation laid by DeepSeek-V3-Base. Both variations offer an impressive context length of up to 32,000 tokens, ensuring comprehensive coverage of mathematical problem-solving scenarios.
Innovative Approach
Delving into the intricacies of the training processes, the developers opted for a revolutionary cold-start training system designed to prompt the model to break down intricate problems into manageable subgoals. These subgoals, once resolved, are seamlessly integrated into the CoT, leveraging the base model’s reasoning abilities to kickstart reinforcement learning. This innovative approach sets the stage for a dynamic and adaptive learning experience that continuously refines the model’s problem-solving capabilities.
Summary
In a bid to democratize access to this cutting-edge technology, DeepSeek has made DeepSeek-Prover-V2 available for download not only on GitHub but also on their Hugging Face listing. By embracing an open-source model release strategy, DeepSeek underscores its commitment to fostering collaborative research and development within the AI community. Although specific details regarding the model’s core architecture and dataset remain undisclosed, the iterative improvements made to its training processes stand out as a testament to the power of continuous innovation in enhancing AI model performance and specialization.
LEAD GENERATION SERVICES FROM APPSREAD

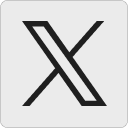




